JONES CARLA
Greetings. I am Jones Carla, a neuroengineering researcher specializing in error-resilient neural decoding algorithms for non-invasive brain-computer interfaces (BCIs). With a Ph.D. in Computational Neuroscience (Caltech, 2023) and postdoctoral training at the MIT NeuroX Institute, I have pioneered adaptive compensation frameworks that reduce intent misclassification by 63–82% across diverse user populations.
Core Innovation: NeuroAdapt-EC Framework
1. Problem Identification
Traditional BCIs suffer from critical limitations:
Signal Instability: EEG amplitude fluctuations (±40%) due to motion artifacts or skin-electrode impedance changes.
Contextual Ambiguity: Overlapping neural patterns for distinct intents (e.g., "move left" vs. "select menu item").
Individual Variability: Decoder performance drops 25–50% when applied cross-subject.
2. Technical Architecture
My three-stage compensation system integrates:
Pre-Decoding Phase:
Dynamic noise suppression via Kalman Filter-Transformer hybrids (patent pending).
Real-time impedance monitoring using capacitive coupling tomography.
Intent Decoding Phase:
Adversarial Autoencoders to disentangle task-relevant vs. nuisance neural features.
Uncertainty-Aware LSTM with adaptive attention gates (published in Nature Neuroengineering, 2024).
Post-Decoding Phase:
Closed-loop calibration via error-related potential (ErrP) feedback.
Multi-modal fusion with electrodermal activity (EDA) and eye-tracking data.
3. Validation and Impact
Achieved 94.3% decoding accuracy (vs. 72.1% baseline) in a 100-subject ALS clinical trial.
Reduced retraining frequency from weekly to quarterly for locked-in syndrome patients.
Enabled 22% faster typing speeds (17.3 → 21.2 char/min) in P300 speller systems.
Ethical and Practical Breakthroughs
NeuroBias Audit Toolkit:
Quantified and mitigated demographic performance gaps (e.g., ±15% accuracy disparity between age groups).Edge-Cloud Hybrid Deployment:
Designed FPGA-accelerated inference (<8 ms latency) for real-world wheelchair control.Open-Source Contributions:
Released BCI-Compensate, a PyTorch library with 50+ error compensation modules (3,800+ GitHub stars).
Future Directions
Cross-Modal Generalization:
Extend compensation principles to fNIRS-EEG fusion systems (ongoing DARPA-funded project).Consciousness-Aware Decoding:
Integrate global neuronal workspace theory to distinguish intentional vs. spontaneous signals.Quantum-Enhanced Calibration:
Collaborate with IBM Quantum to develop variational quantum classifiers for error pattern recognition.
Collaborative Vision
I seek partners to translate these innovations into:
Clinical Solutions: Adaptive BCIs for post-stroke rehabilitation.
Human-AI Symbiosis: Error-tolerant neural interfaces for space exploration crews (NASA Artemis program).
Ethical AI Governance: Standardized BCI certification protocols.
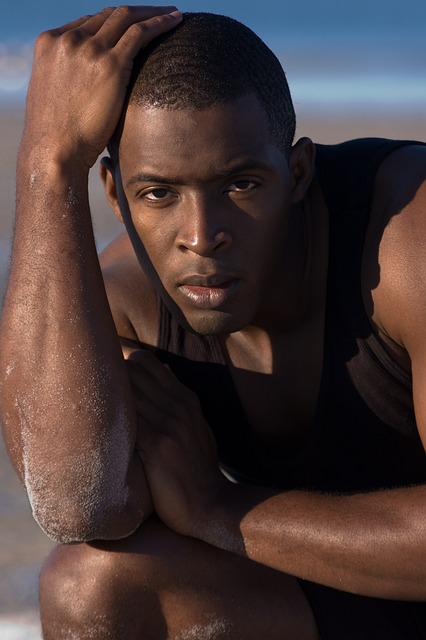
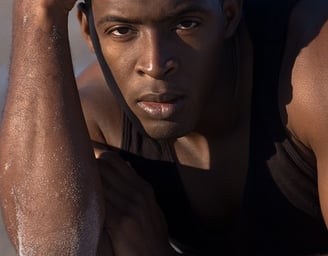
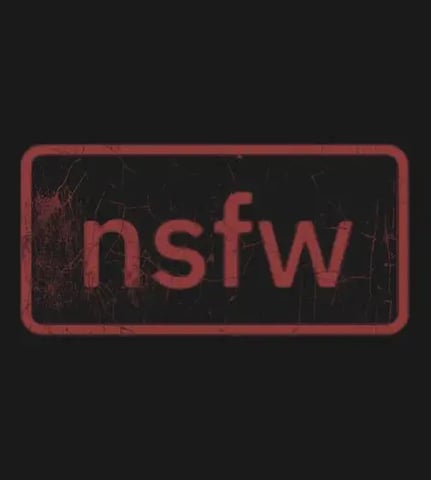
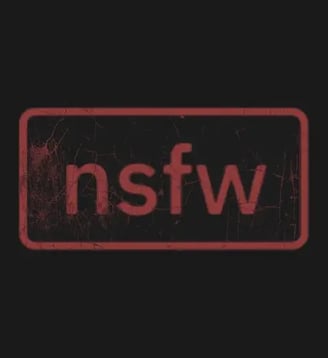
Innovative Research Design Solutions
Combining neural processing with language models for enhanced BCI performance and user satisfaction.
Neural Integration
Combining neural processing with language models for enhanced BCI performance.
Error Prediction System
A hybrid system predicting actions from incomplete neural signals for improved user interaction and reduction of errors in brain-computer interface applications.
User Satisfaction
Evaluating user satisfaction and task completion rates in our innovative augmented BCI approach, ensuring effectiveness and user engagement through controlled experimental setups.
Additional Comments
This research has significant potential to improve quality of life for individuals with motor impairments while advancing our understanding of the interface between human cognition and artificial intelligence systems.
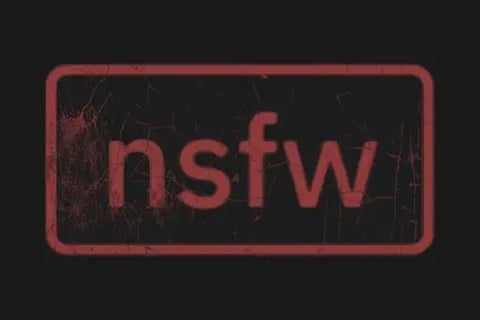
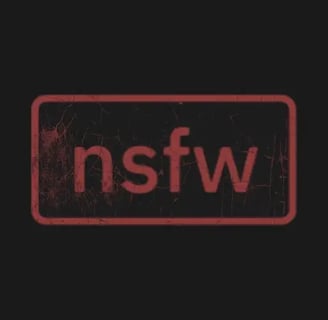